Windstorm losses in Europe – What to gain from damage datasets
Windstorms are among the major natural hazards affecting Western and Central Europe, regularly causing damage to natural and human-made environments, e.g. by uprooting trees or disrupting the power supply. Information on impacts and losses are essential for assessing the windstorm risk and developing adaptation and mitigation strategies. There are different types of datasets that provide this kind of information – from indices that combine meteorological data and insurance aspects, to natural disaster databases, to insurance data based on actual loss reports from insurance companies. But how comparable are these datasets? And what can we learn from the provided information?
To answer these questions, we compare loss reports and estimates from five datasets: PERILS (insurance data), EM-DAT (natural disaster database), XWS, C3S and LI3D (meteorological indices). In particular, we examine differences and similarities in terms of reported events, the number of storms per winter season and the ranking of specific events in 21 European countries.
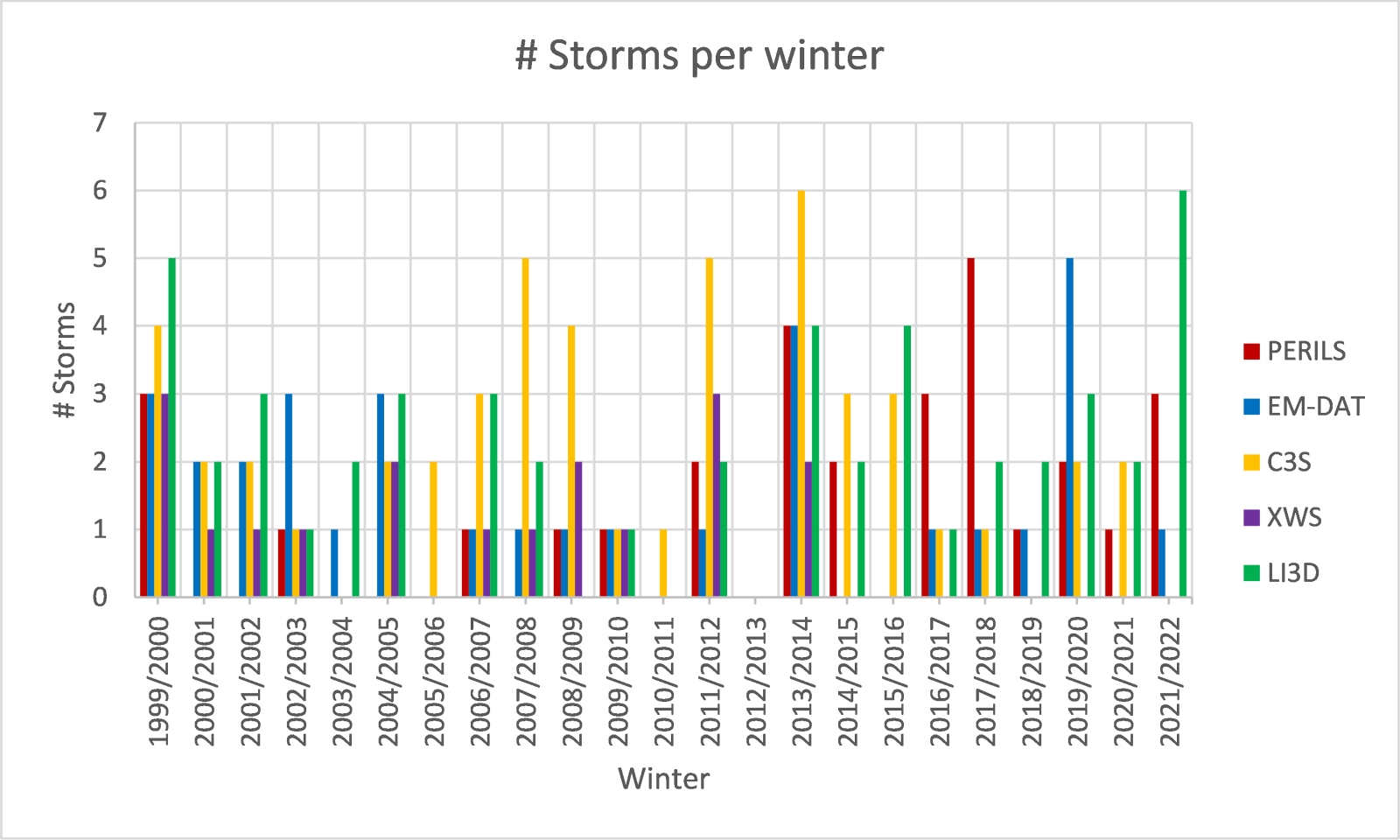
For the period October 1999 to March 2022, a total of 94 storm events were reported: 56 of these events (~ 60%) occurred only in single datasets, while no more than 11 events (< 15%) were documented in all datasets. Therefore, the datasets generally disagree on the number of damaging storms per winter season (see Fig. 1) as well as on the average storm frequency. Numbers are usually higher for the meteorological indices (especially C3S and LI3D) than for the insurance data. Moreover, the ranking of specific storm events varies between the datasets at both European and country level (see Fig. 2). Only individual, typically very strong events such as Kyrill in 2007 show a comparable ranking in the different datasets. Nevertheless, the rank differences can be reduced by focusing on the common events in the datasets.
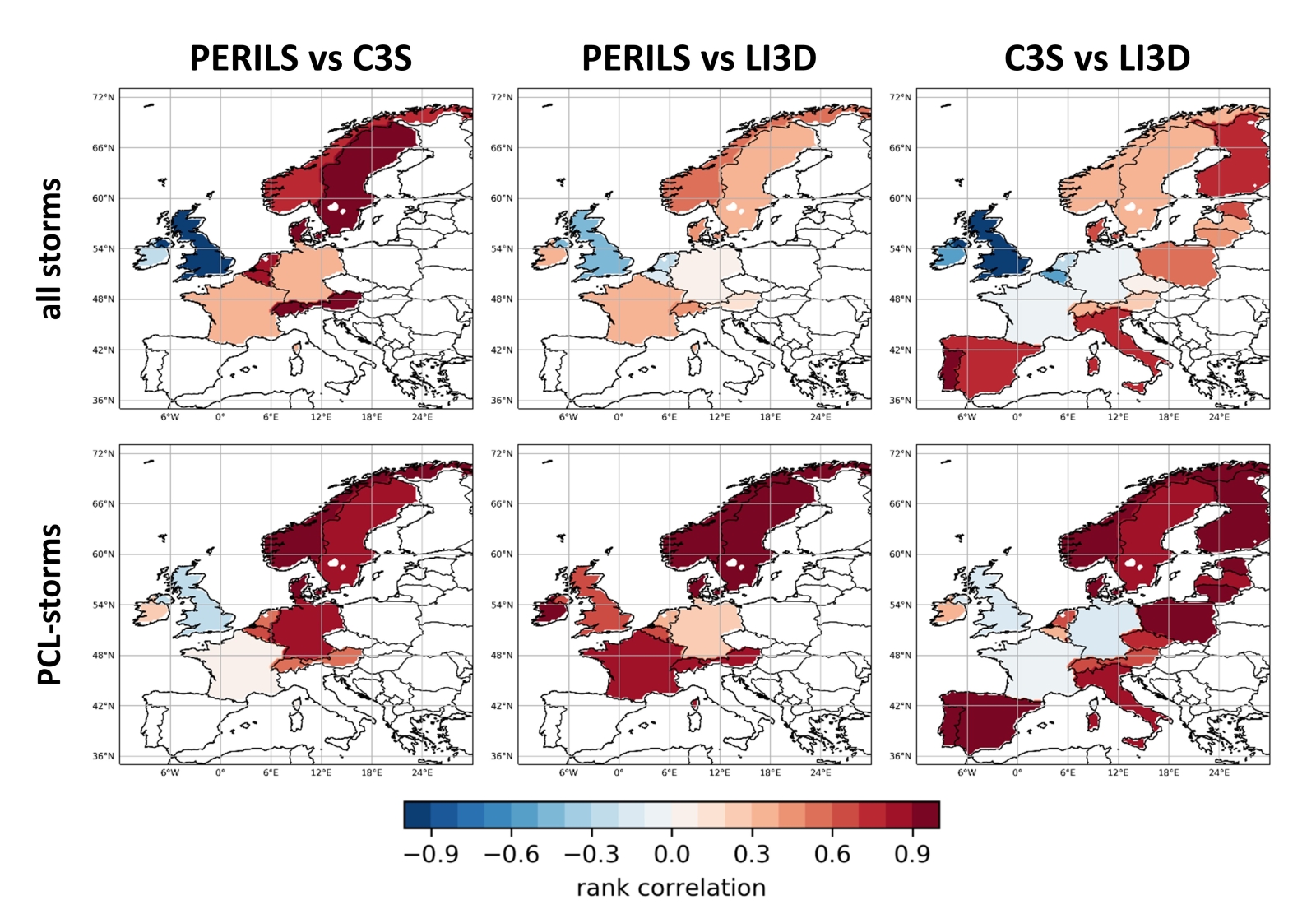
In general, the datasets provide different perspectives on windstorm impacts in Western and Central Europe. This could be used, for example, to assign an uncertainty range to windstorm losses or to test which features are relevant for the calibration of windstorm models. We conclude that only a combination of different datasets can provide a representative picture of windstorm impacts.
The study was presented at a press conference at EGU 2024: https://www.youtube.com/watch?v=YEWnBalFV_o
Moemken J, Messori G, Pinto JG (2024) Windstorm losses in Europe – What to gain from damage datasets. Weather and Climate Extremes, 44, 100661, https://doi.org/10.1016/j.wace.2024.100661.
Julia Mömken and Joaquim Pinto, WG “Regional Climate and Weather Hazards”