Artificial intelligence helps distinguish high-wind areas in winter storms
Wintertime low-pressure systems are often associated with strong winds and precipitation. The former are usually concentrated in certain areas of the low. In particular, the so-called warm jet ahead of the cold front, the cold jet ahead of the warm or occlusion front, convection along the cold front and the area behind the cold front are frequently associated with strong wind and gust speeds. These features have different meteorological characteristics and occur at certain times relative to the cyclone's lifecycle. This suggests that they can be forecasted to a different extent.
Exactly these differences were used by a team around IMK-TRO PhD student Lea Eisenstein to objectively identify the different areas of high winds [1]. “The identification turned out to be more challenging than expected”, says Eisenstein. “This is due to the considerable differences from storm to storm and partly also the influence of the inhomogeneous surface.” In collaboration with Benedikt Schulz, a Mathematics PhD student, an adequate solution was found. The key is – as often in current research – the usage of artificial intelligence, in this case a probabilistic random forest.
For the training of the random forest, the features were first identified subjectively for 12 case studies using station observations. The training is based on only eight meteorological variables: pressure, potential temperature, precipitation, wind speed, wind direction, as well as the hourly tendencies of pressure, potential temperature and wind direction. Both the wind speed and the potential temperature were normalised by a climatology to remove diurnal and seasonal cycles and station-specific effects.
“It turns out that the random forest can learn physically meaningful patterns from the data”, Schulz elaborates. “For example, the pressure tendency tells us whether we are ahead of or behind the cold front, while precipitation is an important sign of convection along the cold front and the pressure indicates how close we are to the cyclone centre.” In the end, the random forest returns the probability of the occurrence of each feature at every station and for every time step. To obtain areal information from the irregularly distributed stations, the probabilities are interpolated over Europe. Figure 1 shows an example for storm Burglind (03/01/2018), more time steps and cases are available at [2].
The software of the new method is publicly available under the name RAMEFI (Random-forest based mesoscale wind-feature identification) [3] and can be used by other researchers. Once trained, the random forest can easily be applied to other datasets, such as reanalyses and model forecasts, independently from the respective resolution. Only the eight parameters listed above need to be available in hourly resolution.
“Overall, we can identify the strong wind features well using a relatively simple approach based on only a few parameters”, summarises Eisenstein, “although the distinction remains difficult in some unusual cases, e.g., for secondary fronts.” The next big challenge now is to evaluate to which extent the forecast errors in the different areas vary and, therefore, can be corrected separately using statistical methods.
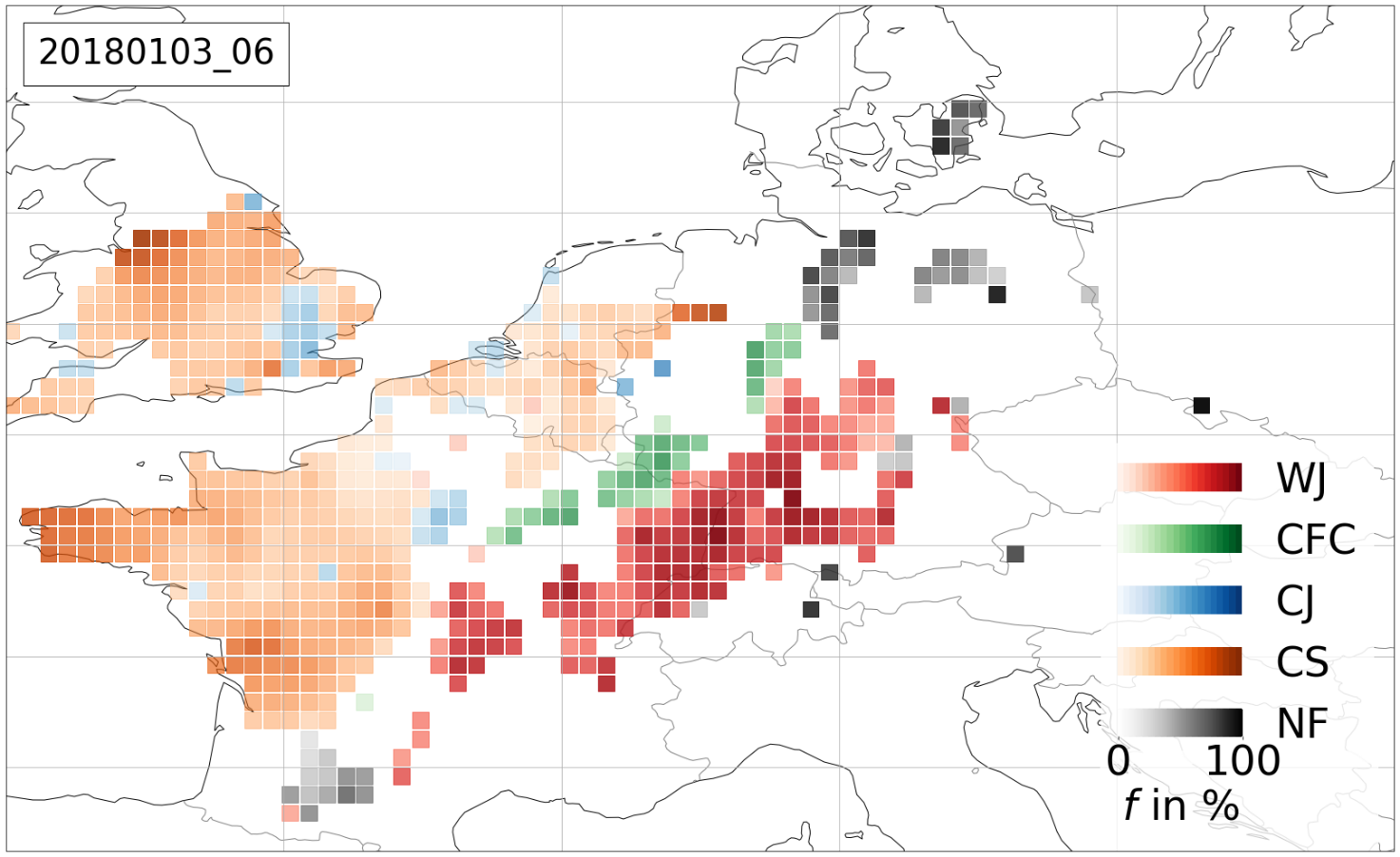
[1] Eisenstein, L., Schulz, B., Qadir, G. A., Pinto, J. G., and Knippertz, P.: Objective identification of high-wind features within extratropical cyclones using a probabilistic random forest (RAMEFI). Part I: Method and illustrative case studies, Weather Clim. Dynam. Discuss. [preprint], https://doi.org/10.5194/wcd-2022-29, in review, 2022.
[2] Eisenstein, L., Schulz, B., Qadir, G. A., Pinto, J. G., and Knippertz, P.: Objective identification of high-wind features within extratropical cyclones using a probabilistic random forest. Part I: Method and illustrative case studies - Video Supplement, https://doi.org/10.5281/zenodo.6541277, 2022.
[3] Eisenstein, L.., Schulz, B., Qadir, G. A., Pinto, J. G., and Knippertz, P.: RAMEFI (RAndom-forest based MEsoscale wind Feature Identification), https://doi.org/10.5281/zenodo.6541303, 2022a.
Working Group: "Atmospheric Dynamics"