Better weather forecasts thanks to artificial intelligence?
Decade-long developments of operational weather forecast systems at national and international weather services (e.g. German Weather Service DWD, European Centre for Medium-Range Weather Forecasts ECMWF) have led to a remarkable increase in forecast quality. Today, for example, tropospheric pressure and temperature values can be predicted for the next 7 days as accurately as for the next 4 days in the 1980s [1]. With the increasing number of extreme weather events due to climate change [2] and the expansion of renewable energy, reliable weather forecasts for days and weeks in advance become more and more important to optimize planning and reduce potential damage. While complex weather forecasting systems have so far been developed based on physical principles, a fundamental paradigm shift is currently taking place: The huge amounts of data from meteorological measuring systems and models, as well as the enormous capability of supercomputers, allow methods of artificial intelligence to be applied to weather forecasting. The ECMWF, for example, has developed an ambitious 10-year plan for this [3].
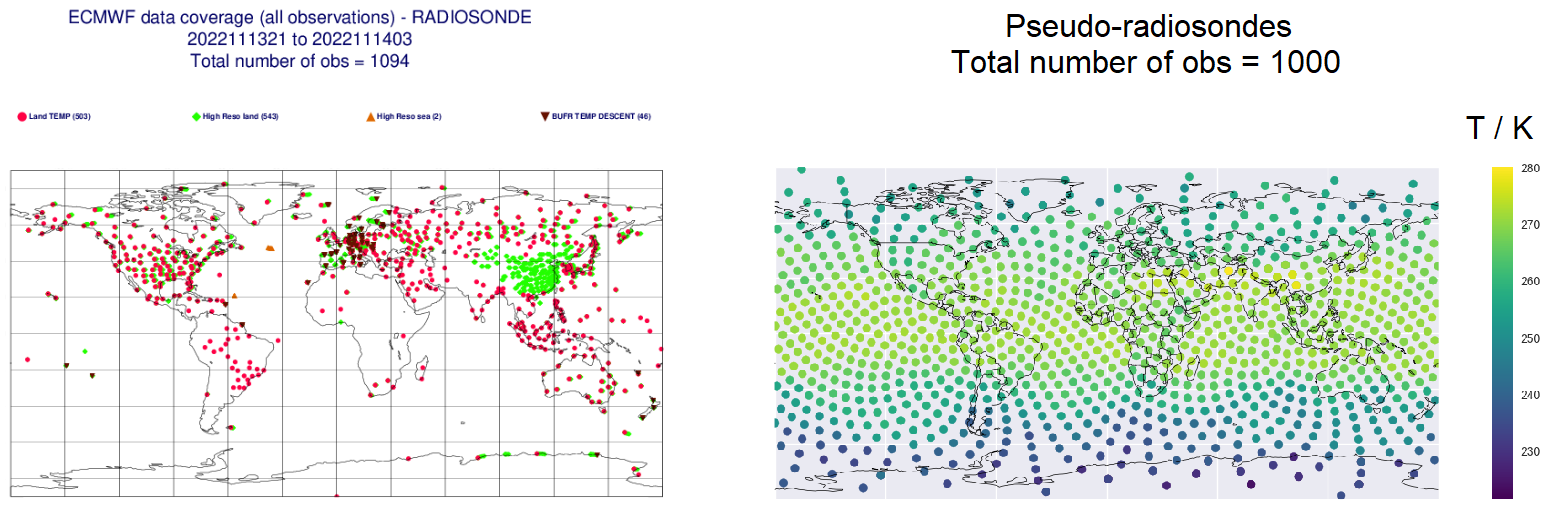
A team of researchers of the KIT project TEEMLEAP (A new TEstbed for Exploring Machine LEarning in Atmospheric Prediction), headed by Prof. Dr. Peter Knippertz (IMK-TRO), has now established a "playground" for investigating the benefits of artificial intelligence along the weather forecast chain on KIT’s high-performance computer HoreKa. They work closely with DWD and use its operational system. On 01 December 2022, a workshop was held at KIT, where the TEEMLEAP team, leading DWD specialists and other national and international experts from the fields of weather observation data, modelling, mathematics, computer science and hydrology discussed the diverse application possibilities of this novel forecast environment. "The testbed allows us to investigate exciting questions along a simplified but realistic forecast chain," explains Dr. Julian Quinting (IMK-TRO). "For example, would better observational data or better forecast models improve the quality of predictions most? Where is the benefit of artificial intelligence greatest?"
To answer these and other questions, global forecast cycles for past weather situations will be simulated, incorporating so-called pseudo-observation data. "We use high-resolution reanalysis data from the ECMWF, which allows us to raise fictitious weather balloons at any location on the globe," explains Dr. Jannik Wilhelm (IMK-TRO). "In contrast to the real observation network, the weather forecast model of DWD thus receives information about the vertical structure of the atmosphere that is otherwise not available everywhere for weather forecasting." (cf. Fig. 1). By changing the accuracy and spatial distribution of the pseudo-observations and varying properties such as their availability and the model resolution, the team can draw conclusions about the importance of the individual components of the forecast chain.
In addition, the experiments can be used to further develop machine learning methods for the statistical post-processing of weather forecasts [4]. "Due to the complexity of the physical system of the atmosphere, it is crucial to estimate the probability of weather events such as storms or heat waves as optimally as possible. We have recently demonstrated that artificial intelligence has a huge potential for the post-processing of weather forecasts," says TEEMLEAP scientist and junior research group leader Dr. Sebastian Lerch (ECON).
In combination with a cost estimate for possible improvements of the observation and model systems, the results of TEEMLEAP's investigations can support weather services in their strategic decisions for the further development of operational systems and provide valuable new methods for application in the forecast chain.
[1] T. Haiden, M. Janousek, F. Vitart, Z. Ben Bouallegue, L. Ferranti, F. Prates (2021): Evaluation of ECMWF forecasts, including the 2021 upgrade. Technical memorandum. http://dx.doi.org/10.21957/90pgicjk4
[2] IPCC (2018): Global Warming of 1.5°C. An IPCC Special Report on the impacts of global warming of 1.5°C above pre-industrial levels and related global greenhouse gas emission pathways, in the context of strengthening the global response to the threat of climate change, sustainable development, and efforts to eradicate poverty [V. Masson-Delmotte, P. Zhai, H.-O. Pörtner, D. Roberts, J. Skea, P.R. Shukla, A. Pirani, W. Moufouma-Okia, C. Péan, R. Pidcock, S. Connors, J.B.R. Matthews, Y. Chen, X. Zhou, M.I. Gomis, E. Lonnoy, T. Maycock, M. Tignor, and T. Waterfield (eds.)]. Cambridge University Press, Cambridge, UK and New York, NY, USA, 616 pp. https://doi.org/10.1017/9781009157940
[3] P. Dueben, U. Modigliani, A. Geer, S. Siemen, F. Pappenberger, P. Bauer, A. Brown, M. Palkovic, B. Raoult, N. Wedi, V. Baousis (2021): Machine learning at ECMWF: A roadmap for the next 10 years. Technical memorandum. http://dx.doi.org/10.21957/ge7ckgm
[4] S. Vannitsem, J.B. Bremnes, J. Demaeyer, G.R. Evans, J. Flowerdew, S. Hemri, S. Lerch, N. Roberts, S. Theis, A. Atencia, Z. Ben Bouallègue, J. Bhend, M. Dabernig, L. De Cruz, L. Hieta, O. Mestre, L. Moret, I.O. Plenković, M. Schmeits, M. Taillardat, J. Van den Bergh, B. Van Schaeybroeck, K. Whan, J. Ylhaisi (2021). Statistical Postprocessing for Weather Forecasts: Review, Challenges, and Avenues in a Big Data World, Bulletin of the American Meteorological Society, 102(3), E681-E699. Retrieved Nov 14, 2022, from https://journals.ametsoc.org/view/journals/bams/102/3/BAMS-D-19-0308.1.xml